Veículos aéreos não tripulados e sensores remotos: ferramentas para o gerenciamento dos recursos florestais
DOI:
https://doi.org/10.20873/jbb.uft.cemaf.v8n2.vsouzaPalavras-chave:
sensoriamento remoto, VANTs, vegetaçãoResumo
O objetivo deste trabalho é descrever, através de revisão da literatura, o uso dos sensores remotos integrados aos Veículos Aéreos Não tripulados (VANT). Os tipos sensores e seu funcionamento também são apresentados além dos métodos de processamento e aplicações na silvicultura e na proteção contra incêndios florestais. O VANT foi utilizado inicialmente em atividades militares, principalmente para atender monitoramento de áreas de difícil acesso, consideradas inósptas para o ser humano. Com o desenvolvimento tecnológico dos veículos equipados com Sistema Globais de Navegação por Satélite (GNSS) e minituarização dos sensores possibilitaram a captação da energia eletromagnética refletida e transmitida pelos alvos na superfície, sendo posteriormente convertida em sinais elétricos de maneira que podem ser armazenadas ou transmitidas em tempo real. Os dados capturados pelos sensores são processados para serem utilizados no processo de aquisição de informações dos objetos observados na superfície terrestre para serem aplicados no gerenciamento dos recursos florestais.
Referências
Andersen HE, Mcgaughey RJ, Reutebuch SE. Estimating forest canopy fuel parameters using LIDAR data. Remote Sensing of Environment, v.94, n.4,p.441-449, 2005.
Bendig J, Yu K, Aasen H, Bolten A, Bennertz S, Broscheit J, Gnyp LM, Bareth G. Combining UAV-based plant height from crop sufarce models, visible and near infrared vegetation indices for biomass monitoring in barley. International Journal of Applied Earth Observation and Geoinformation, v.39, p.79-87, 2015.
http://doi.org/10.1016/j.jag.2015.02.012.
Colomina I, Molina P. Unmanned aerial systems for photogrammetry and remote sensing: A review. ISPRS Journal of Photogrammetry and Remote Sensing, v.92, p.79-97, 2014.
https://doi.org/10.1016/j.isprsjprs.2014.02.013.
Geipel J, Peteinatos GG, Claupein W, Gerhards R. Enhancement of Micro Unmanned Aerial Vehicles for Agricultural Aerial Sensor Systems. In Precision Agriculture ’13, Ed.J. V. Stafford, p. 161-167. Wageningen, the Netherlands: Wageningen Academic Publishers, 2013.
Getzin S, Wiegand K, Schöning I. Assessing biodiversity in forests using very high‐resolution images and unmanned aerial vehicles. Methods in Ecology and Evolution, v.3 n.2, p.397-404, 2012.
https://doi.org/10.1111/j.2041-210X.2011.00158.x
Giongo M, Koehler HS, Viola MR, Bastos OS, Santos AF, Santopuoli G. Estimativa da altura da base das copas com o uso de dados laser scanning aerotransportado (LiDAR). Journal of Biotechnology and Biodiversity, v.3, n.3, p.48-57, 2012.
https://doi.org/10.20873/jbb.uft.cemaf.v3n3.giongo
Gitelson AA, Kaufman JY, Stark R, Rundquist D. Novel algorithms for remote estimation of vegetation fraction. Remote Sensing of Environment, v.80, n.01, p.76–87, 2016.
https://doi.org/10.1016/S0034-4257(01)00289-9
Hardin PJ, Jensen RR. Veículos aéreos não tripulados de pequena escala em sensoriamento remoto ambiental: desafios e oportunidades”. GIScience e sensoriamento remoto, v.48, n.1, p.99-111, 2011.
https://doi.org/10.2747 / 1548-1603.48.1.99
Helbich M. Spatiotemporal Contextual Uncertainties in Green Space Exposure Measures: Exploring a Time Series of the Normalized Difference Vegetation Indices. Int. J. Environ. Res. Public Health, v.16, n.05, p.852, 2019.
https://doi.org/10.3390/ijerph16050852
Huete AR, Didan K, Miura T, Rodriguez PE, Gao X, Ferreira LG. Overview of the radiometric and biophysical performance of the MODIS vegetation indices. Remote Sensing of Environment, v.83, n.01, p.195-213, 2002.
https://doi.org/10.1016/S0034-4257(02)00096-2
Jensen JR. Sensoriamento Remoto do Ambiente: Uma perspectiva em recursos terrestres. São José dos Campos, SP: Parêntese, 2009.
Jorge LAC, Namasu RY. Uso de veículos aéreos não tripulados (VANT) em agricultura de precisão. Embrapa Instrumentação-Capítulo em livro científico (ALICE), 2014.
Kataoka T, Kaneko T, Okamoto H, Hata S. Crop growth estimation system using machine vision. Proceedings 2003 IEEE/ASME International Conference on Advanced Intelligent Mechatronics (AIM 2003), v.02, n.01, p.1079-1083, 2003.
http://doi.org/10.1109/AIM.2003.1225492
Key CH, Benson NC. Measuring and remote sensing of burn severity. In: Neuenschwander LF, Ryan KC. Proceedings Joint Fire Science Conference and Workshop. Moscow: 3ed. University of Idaho and International Association of Wildland Fire. 1999, p. 284.
Kurihara J, Ishida T, Takahashi Y. Unmanned Aerial Vehicle (UAV)-based hyperspectral imaging system for precision agriculture and forest management. In Unmanned Aerial Vehicle: Applications in Agriculture and Environment (p. 25-38). Springer, Cham, 2020
Li W, Niu Z, Chen H, Li D, Wu M, Zhao W. Remote estimation of canopy height and aboveground biomass ofmaize using high-resolution stereo images from a low-costunmanned aerial vehicle system. Ecological Indicator, v.67, n.01, p.637-648, 2016.
http://dx.doi.org/10.1016/j.ecolind.2016.03.036
Liu WTH. Aplicações de sensoriamento remoto. Campo Grande: Oficina de Textos, 2015. 908p.
Lopes BA, Silva MM. Estratégias funcionais de Vanilla chamissoni (Orchidaceae) em ambiente arbustivo e florestal de restinga. Revista Brasileira de Geografia Física, v.12, n.02, p.355-364, 2018.
https://doi.org/10.26848/rbgf.v12.2.p355-364
Mapir camera. Calibration Target (V2) capture Procedure. 2020. Disponível em: < https://www.mapir.camera/pages/calibration-target-capture-procedure-v2/>. Acesso em: 20 fev. 2020.
Meinen BU, Robinson DT. Mapping erosion and deposition in an agricultural landscape: Optimization of UAV image acquisition schemes for SfM-MVS. Remote Sensing of Environment, v.239, n.111666, 2020.
https://doi.org/10.1016/j.rse.2020.111666
Miranda V, Pina P, Heleno S, Vieira G, Mora C, Schaefer CE. Monitoring recent changes of vegetation in Fildes Peninsula (King George Island, Antarctica) through satellite imagery guided by UAV surveys. Science of The Total Environment, v.704, p.135295, 2020.
https://doi.org/10.1016/j.scitotenv.2019.135295
Mohammed HG, Colombo R, Middleton E, Rascher U, Van Der Tol C, Nedbal L, Goulas Y, Pérez – Priego O, Damm A, Meroni M, Joiner J, Cogliati S, Verhoef W, Malenovsky Z, Gastellu – Etchegorry JF, Miller J, Guanter L, Moreno J, Moya I, Berry JA, Frankenber C, Zarco Tejada PJ. Remote sensing of solar-induced chlorophyll fluorescence (SIF) in vegetation: 50 years of progress. Remote Sensing of Environment, v.231, n.01, p.01-38, 2019.
https://doi.org/10.1016/j.rse.2019.04.030
Moraes CE. Formação continuada de professores: curso de astronáutica e ciências do espaço. São Paulo: Editado pelo programa AEB Escolada Agência Espacial Brasileira, 2008. 78p.
Novo EML. Sensoriamento Remoto: princípios e aplicações. São Paulo: Edgard Blucher, 2010. 388p.
Pádua L, Vanko J, Hruška J, Adão T, Sousa JJ, Peres E, Morais R. UAS, sensors, and data processing in agroforestry: A review towards practical applications. International journal of remote sensing, v.38, n.8-10, p.2349-2391, 2017.
https://doi.org/10.1080/01431161.2017.1297548
Pinty B, Verstraete M. GEMI - A nonlinear index to monitor global vegetation from satellites. Vegetation, v.101, n.01, p.15-20, 1992.
Ponzoni JF. et al. Sensoriamento remoto da vegetação. 2. ed. Atualizada e ampliada, São Paulo, SP: Oficina de Textos, 2015. 45p.
Przybilla HJ, Wester-Ebbinghaus W. Bildflug mit ferngelenktem Kleinflugzeug. Bildmessung und Luftbildwesen, v.47, n.5, p.137-142, 1979.
Raj R, Kar S, Nandan R, Jagarlapudi A. Precision Agriculture and Unmanned Aerial Vehicles (UAVs). In: Avtar R., Watanabe T. (eds) Unmanned Aerial Vehicle: Applications in Agriculture and Environment. Springer, Cham.
Rosa R. Introdução ao Sensoriamento Remoto 7.ed. Uberlândia: EDUFU, 2009. 264p.
Rouse W, Haas HR, Schell AJ, Deering WD. Monitoring vegetation systems in the Great Plains with ERT. Washington, NASA: third earth resources technology Satellite-1 symposium, 1 ed. 1974, 309p.
Shahrokhnia HM, Ahmadi HS. Remotely Sensed Spatial and Temporal Variations of Vegetation Indices Subjected to Rainfall Amount and Distribution Properties. Spatial Modeling in GIS and R for Earth and Environmental Sciences, v.01, n.01, p.21-53, 2019.
https://doi.org/10.1016/B978-0-12-815226-3.00002-8
Simelli I, Tsagaris A. The Use of Unmanned Aerial Systems (UAS) in Agriculture. In HAICTA(p. 730-736).
Soares VR. et al. Incêndios florestais. Curitiba: UFPR, 2017. 255 p.
Souza VI, Santos MM, Giongo M, Carvalho VE, Machado SEI. Estimativa do material combustível em área de cerrado campo sujo a partir de imagens do sensor RGB. Pesquisa Florestal Brasileira, v.3, n.1, p.01-06, 2018.
https://doi.org/10.4336/2018.pfb.38e201801706
Taiz L, Zeiger E, Moller MI, Murphy A. Fisiologia e Desenvolvimento Vegetal. 6. ed. Porto Alegre, RS: ARTMED EDITORA LTDA, 2017. 731p.
Verhoeven G. Taking computer vision aloft – Archaeological three-dimensional reconstructions from aerial photographs with PhotoScan. – Archaeological Prospection, v.18, n.1, p.67-73, 2011.
Vieira LE, Souza SG, Santos RA, Silva S.J. Manual de fisiologia vegetal. 1. ed. São Luis, MA: EDUFMA, 2010. 230p.
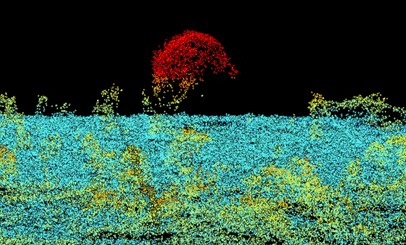
Publicado
Como Citar
Edição
Seção
Licença
Copyright (c) 2024 - Journal of Biotechnology and Biodiversity
Este obra está licenciado com uma Licença Creative Commons Atribuição 4.0 Internacional.
Autores que publicam nesta revista concordam com os seguintes termos:
Autores mantêm os direitos autorais e concedem à revista o direito de primeira publicação, com o trabalho simultaneamente licenciado sob a Licença Creative Commons Attribution (CC BY 4.0 no link http://creativecommons.org/licenses/by/4.0/) que permite o compartilhamento do trabalho com reconhecimento da autoria e publicação inicial nesta revista.
Autores têm autorização para assumir contratos adicionais separadamente, para distribuição não exclusiva da versão do trabalho publicada nesta revista (ex.: publicar em repositório institucional ou como capítulo de livro), com reconhecimento de autoria e publicação inicial nesta revista.
Autores têm permissão e são estimulados a publicar e distribuir seu trabalho online (ex.: em repositórios institucionais ou na sua página pessoal) a qualquer momento antes ou durante o processo editorial, já que isso pode gerar alterações produtivas, bem como aumentar o impacto e a citação do trabalho publicado (disponibilizado em O Efeito do Acesso Livre no link http://opcit.eprints.org/oacitation-biblio.html).